Brian Scott
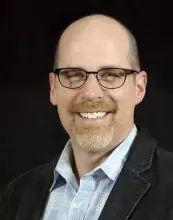
Brian Scott, president and founder of ClearTone Consulting LLC, provides executive technology consulting services based on 35 years of technology expertise and 20 years of exhibitions and events industry experience.
In my last blog post, I discussed how several virtual platforms and standalone matchmaking services tout the promise of highly effective matching between attendees to attendees as well as attendees to exhibitors. In reference to the data that is available to any matchmaking system and the subsequent value that’s likely to be output, I dug up that age-old saying “garbage in, garbage out.”
There are also more than a few vendors out there that love to put the ”AI” label on their process as if to assure event organizers that just because artificial intelligence is being used that highly accurate matches will be guaranteed. Unfortunately, it simply does not work this way.
I promised that I’d suggest some approaches that event organizers could take to their respective platform providers to better the effectiveness of the matchmaking, but before I do, let me explore some simple use cases to explain why many event organizers are not having positive experiences in this area.
I’ll use a hypothetical attendee as an example. Let’s name him Herbert. Herbert attended a virtual meeting and completed a registration process, where he was asked his name and other basic profile information such as email, etc. He was also then presented with a few demographic questions. The first question asked for his title and the second question asked for his company name. That’s all we know about Herbert other than he has a warm and inviting name.
Herbert happens to be in the human resources department of a small company named Acme. Given its focus on employee wellbeing, Acme recently created new titles for HR team members and Herbert’s new moniker is chief motivational officer. Acme was also purchased last year by a billion-dollar company and is partially integrated in areas such as procurement and back-office systems, but retained its name and market autonomy.
If the registration information is all we knew about Herbert, it would be easy to classify him as a C-level executive for a small company. Without more information, matchmaking systems may have him meet with other executives, positioned as a top decision-maker or buyer, and limited to small business groups. The reality is that he’s not a buyer or executive, and is actually part of a much larger organization.
The first session Herbert visited didn’t help the situation. He recently became particularly concerned about retention in Acme’s technology department, so he attended a session in the “Tech” track to better understand the mindset and environmental influences. This led the matchmaking algorithms to believe that Herbert is a technologist and should be matched with other techie attendees.
It’s pretty easy to understand how the matchmaking system didn’t have a lot to go on and was simply not effective. This is where a much more strategic data capture plan that is meant to specifically support effective matches could play a very positive role.
To continue this example, what if we asked Herbert to identify his specific department, to prioritize his top two reasons to attend the meeting, to enter his top three products that he may have interest in and to list his top three personal interests? As you can imagine, this data could really drive matching algorithms and greatly increase the odds of making meaningful connections.
But there’s a challenge with these types of questions. In each case, there could be many options available for him to select. How many departments do you list to try to cover them all? How many reasons to attend? And the big one: How many products do you have to list? Making so many choices available with checkboxes next to them is an archaic way of approaching user website interfaces and would likely result in an aggravated user. This is where new technology such as AI can easily help.
Instead of the multiple columns of lists with checkboxes, what if there was just a text box for Herbert to enter anything he wanted? This certainly makes it easy for Herbert but results in an unstructured data set on the backend. Under department, he could enter “Human Resources,” “HR,” “People and Development,” “Employee Wellbeing” — or any number of options (including misspellings).
A good implementation of artificial intelligence can read these entries, understand what was intended, account for misspellings and categorize them in a structured way. Now we have a rich, structured data set on the backend to drive all kinds of great matches and still have a simple, easy-to-use interface for the attendee.
Another option would be for the systems to execute basic screen scrapes off the website associated with the email address domains and use that data to create an “interest” picture of the attendee. It’s fairly straightforward today to programmatically understand a great deal about a company from just analyzing the content available on the site. This content spans products, services, company culture and values, industry, the kinds of positions that may be open, ownership...the list goes on and on.
Between these two, there is significantly more data specific to an attendee focused on understanding who they are and what they’re interested in. I’m highly confident that with deep data such as this, any matchmaking service worth it’s weight in salt would be able to produce phenomenal connections that resonate with the attendees and exhibitors and increase the value and ROI of your meeting. I suppose the opposite of “garbage in, garbage out” is “treasure in, value out” and that’s exactly what we all want for our stakeholders.